Events
Explainable Machine Understanding and Examples for Clinical Applications - Lecture by Alexander Löser
This article is about Alexander Lösers presentation that he gave at the AI Business Meetup on January 28th 2020 at the WeWork Sony Center.
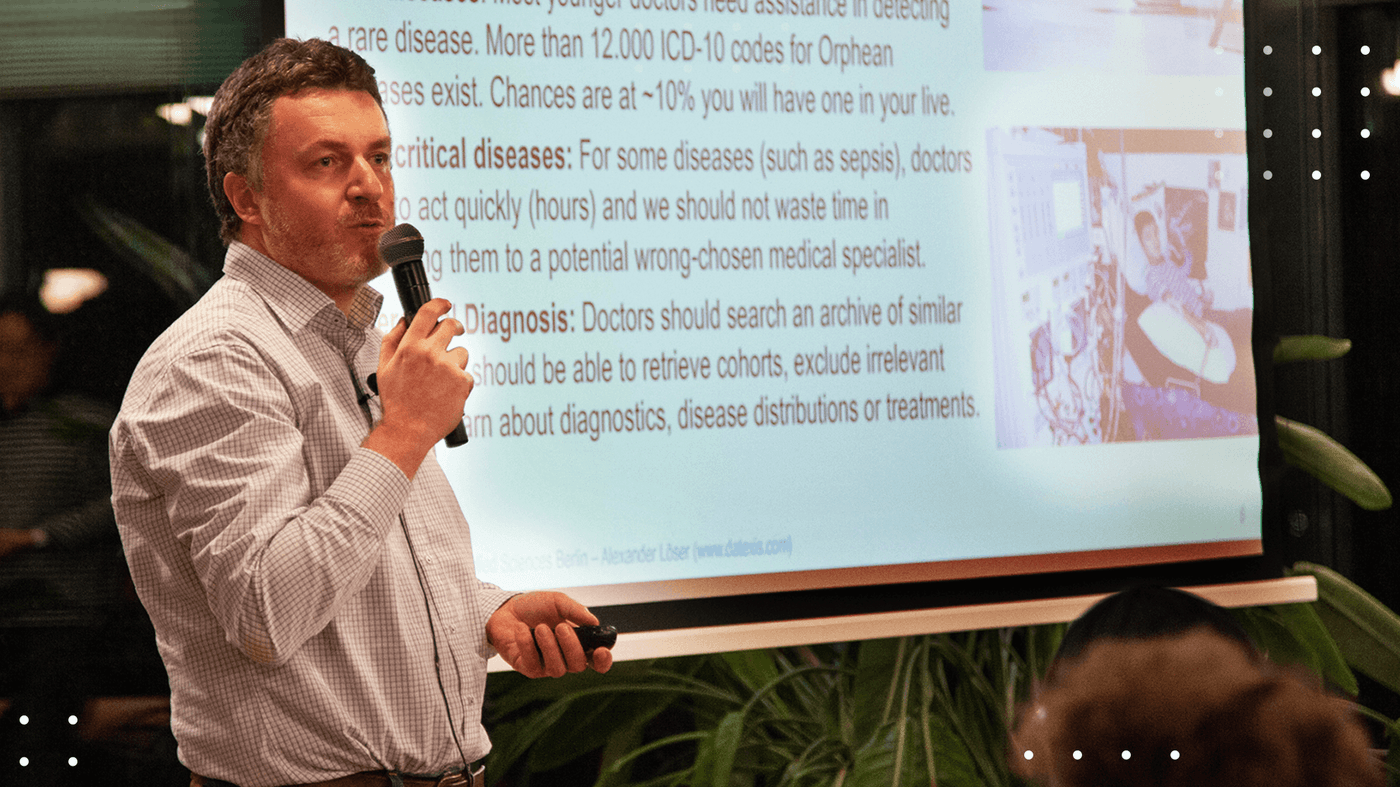
Alexander Löser, professor at the Beuth Hochschule für Technik Berlin, has been a public officer for 25 years. He therefore represents universities, industries and the “Learning System” platform, which comes from the Ministry of Research. Together with a group of ten colleagues, including PhD students, he is working on research on the subject of machine understanding (WWW.DATEXIS.COM), to which he joined the AI Business Meetup. He gave a talk at the WeWork Sony Center on January 28th 2020.
Europe and Africa on the same level
Löser talked about Europe’s current economic situation. In the past few years, economy has developed positively, yet Europe is on the same level as Africa, considering market capitalization and goodwill. In the year 2018, Germany’s growth product was 3,9 billion US-Dollars. Companies such as Huawai record just this though. In the USA, there are multiple companies that drive forward not only this growth product, but also the United States in general. That just shows that Germany has to do something.
The solution would be entering protected markets such as health- or machine industries. Artificial Intelligence as well as efficient technologies have to be supported. According to Löser, the AI meetup should foster this development, since there’s only one Global Player in Europe right now (SAP).
Some facts about health care
For more than four years, doctors have been helped to deal with demographic change. The task is to understand core processes and develop interesting data products that can be used for text and deep learning.
One question that doctors often ask developers is: “Can you narrow down whether it’s a serious illness or an emergency situation or just a little blood on your finger?” The latter is not uncommon at the Charité; only 5-8% of cases are serious illnesses. More than 15,000 rare diseases are only diagnosed after six or seven years! Often the solutions are already written in doctor’s letters, which simply have to be revealed.
Particularly in the case of time-critical diseases, action must be taken quickly. The best way to find information would be for machines to filter and store it from doctor’s letters. Löser adds that differential diagnosis is one of the most important processes in the collection of information.
Clinical use for doctor’s letters
A typical doctor’s letter in Germany is quite easy to recognize: letterhead, address, ending. However, the “main part” consists of the individual expression of the doctor. This may vary from institute to institute and may, for example, contain many abbreviations or no sentences at all. The problem that arises from this is how to find out what is written about at all - whether it is a diagnosis, symptoms or drug treatment.
All these topics, of which there are still thousands, are put into an intelligent language model. In the case of the research group, this model is BERT, a model developed by Google to better understand user search. Löser’s group asked themselves: “Can we make sense of these sentences and classify the subject for each sentence so that it is not just a document classification but a sentence classification?”
From this very question an academic work developed, which was presented at the TACL in 2019. The research group showed an example of the solutions, which looks as follows: A topic is highlighted (topic: therapy) and a doctor’s letter is shown. Dark blue highlighting means that this topic is strongly recognized. Light blue shows that the topic is less well recognized. No color means that it was not recognized. Conservative treatment is thus recognized as a therapy, although it literally has nothing to do with each other - but semantically it does.
If you link all these data products together, you come to the latest publication of the research group. Queries/questions can be queried for entities that can be linked to medical taxonomies.
Demo of a food navigator for food allergies
In the query, the demo is about a food allergy. The aspect to be examined is the treatment. The most relevant records are printed in bold. The words “diseases” and their aspects must first be projected into a separate vector space and then integrated into a common vector space. Afterwards a representation in this common vector space has to be found according to a series of queries, whereby the question arises how exactly these vector spaces can be designed.
Another question arose regarding the query time: If a query against vector space would be hidden, this would be a very expensive query, because it would have to be compared with all existing representations and common principles of research are used with tools like Spotify, Agent SV from Microsoft or other files. It is therefore an elastic search, which is only possible for newer networks.
Another interesting discovery
In November 2019 the research group presented an academic paper in Beijing. This work was done by Betty van Arken and Benjamin Winter, both second year PhD students. The task was to find out what BERT was doing to answer questions and finally to present it.
Common data sets were used, such as the SQuAD of Stanford University, which consists of a set of questions and a set of answers. The measuring accuracy is more than 90%. Then there is bAbI, a benchmark from Facebook, which contains very simple and sometimes “stupid” questions. The third benchmark used is Hotpot QA.
Before a thesis was developed, the researchers wondered what would have been done fifteen years ago if a system such as BERT had been created. First, the sentence would have been extracted and examined for co-references inside or outside. Then one would perform a relational extraction for these potential arguments and later map query types from these extracted relationships to find out if the query type matches a potential answer. In the end, independent components would probably have been created.
Finally, the hypothesis was that BERT could act as such a system and specialise in each layer for some of these tasks. The group then examined five tasks, which are called “exploratory tasks”. These were as follows:
- Entity link
- Co-reference
- Relation extraction
- Question Type
- Extraction of supporting facts
The group discovered that the network is actually doing something similar to what they would have done fifteen years ago. The network uses the distribution hypothesis - the word is described by its neighbors and solved by the entity link.
BERT examines the sample text and tries to find correct answers, which works up to 30%. DATEXIS tries to visualize this process. That’s why they went through all these layers. Basically, the network separates the query and other tokens from a potential answer. DATEXIS. com explains transformer networks. Enter your own text or select another query, then click on the button to forecast and visualize. The first two layers are created very quickly, while the last ones take about 20 seconds.
In the end…
At the end of his presentation, Professor Löser appealed to the role of the government in helping institutes to produce very good AI graduates. The talk was a good introduction to the current situation of AI in Germany and its potential future in the medical field. If you are interested in seeing the whole presentation, you can watch the video here.