Events
OMQ at AI Monday in Berlin
OMQ spoke at AI Monday in Berlin about the automation of customer service by AI and the use of chatbots in shared mobility.
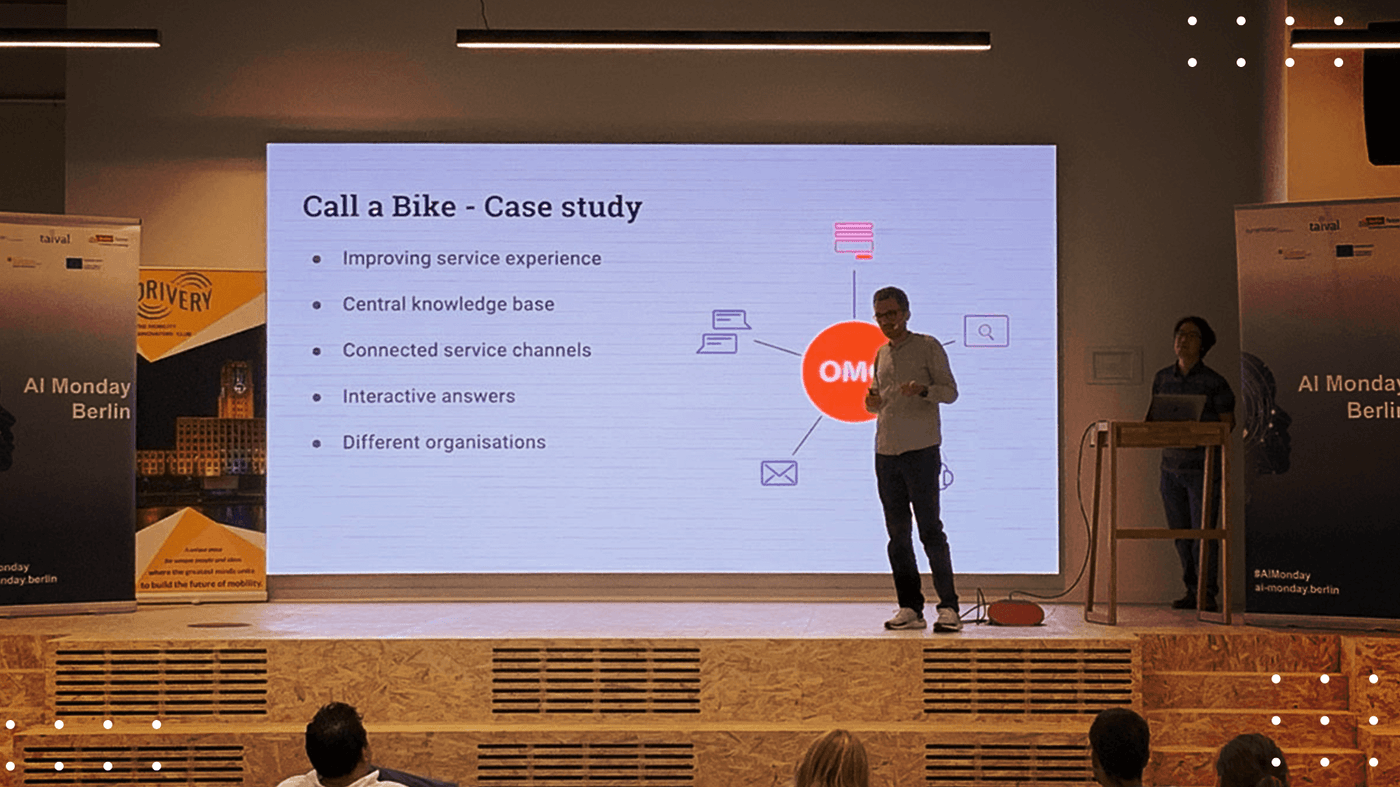
OMQ had been invited to attend AI Monday in coworking space The Drivery in Berlin-Tempelhof on September 2nd, 2019. Our CEO Matthias Meisdrock and Machine Learning Engineer Dr. Tae-Gil Noh explained how OMQ is using Artificial Intelligence in mobility service providers’ customer service in order to improve the customer experience.
Every day, customers and users of mobility services are having negative experiences with customer service that could actually be avoided.
This also happened to our CEO Matthias Meisdrock in New York during a business trip:
Because of poor customer service, I wandered aimlessly around New York for two hours.
said Matthias Meisdrock to introduce the presentation. He had hired a bicycle from Citi Bike. It all worked marvelously, and the return was no problem either. However, when he wanted to use another bike later on, nothing worked any more. He had supposedly not returned the bike correctly. He went onto the Citi Bike website on his smartphone to find a solution on the help page. Unfortunately, he only found an endless list of questions that did not help him and there was no facility for entering his question in a chat.
So the only option left to him was to call the call center. After hanging on for a long time in the call queue, he was finally connected to an employee. Unfortunately, it turned out that that person only had limited access to the system and could not tell him where his bike was.
That meant that he had to walk around New York without proper instruction to try and solve the problem himself. This was an example of very poor customer service.
What customers actually want is to have a positive experience and to have their problem resolved as quickly as possible. Matthias would thus have liked to be able to quickly type in his question and receive an answer immediately. In Matthias’ case, the ideal situation would have been a chatbot, which reports the previous bike was not returned properly and passes the bike’s location using GPS data.
How can AI be used to produce a positive customer experience?
In order to produce a positive customer experience, the customer must receive help directly and fast in the event of a problem. The customer should be well served on any service channel and always receive appropriate solutions. In a chat, for example, the customer receives the same information regarding their problem as when they enter their question on the help page. To make sure that all service channels can access the same knowledge, an intelligent knowledge database is used that contains all the questions and answers and is used by all products. This enables the customer to type in their question directly, for instance on the contact form, and to receive the appropriate answer displayed right next to it in real time. The AI thus automatically proposes suitable solutions, based on the backend information from the database, and the customer has a positive experience. This eliminates long waiting times of two to three days.
Our customer Deutsche Bahn Connect has already been using the OMQ technology since 2013, applying it among other things for Call a Bike, Flinkster, and StadtRad Hamburg with the aim of continuously improving its customer service. With this technology, all service channels of the mobility service provider are connected to the questions and answers from the database.
In the case of the contact form, for instance, questions are entered in real time and resolved for the customer immediately by means of the intelligent technology. Interactive answers, involving, for example, logging directly into the customer account, make it easy for the customer to have positive experiences with customer service.
How is the chatbot being used in customer service?
As a customer with a question, I go onto the website to obtain a solution to my problem. A chat involves a direct conversation where the chatbot acts as a direct contact for the customer who will help them with their problem immediately. Like the other service channels, the chatbot has access to the expert knowledge from the database and can pass it on quickly to the customer.
A chat at StadtRad Hamburg can go something like this:
Customer: Hello, how do I get the key for the bike?
Chatbot: Hello, you can order a key ring for the bike hire...
Please let me have your customer number and you will receive the key through the post.
Customer: My customer number is: 1234567
Chatbot: Thank you very much! You will receive your key shortly.
Based on the information from the back end system that the chatbot accesses, the key ring is then sent to the customer. Totally without manual intervention by a member of staff as this simple solution is provided by a complex technological process taking place in the background.
There is no complex installation involved that would mean extra work and cost for companies. The OMQ Chatbot can be integrated with existing support and chat systems such as LiveZilla or Userlike through simple plug & play.
The AI in the system
What does Artificial Intelligence (AI) mean?
Put simply, Artificial Intelligence is a system that recognizes patterns from various examples on its own. In our case, for instance, it “learns” that the word “bike” is linked to “key” and can therefore assign a suitable question.
To make it more generally understandable, we shall break it down into the most important key components. AI faces a number of complicated challenges, explains our Machine Learning Engineer Dr. Tae-Gil Noh:
The greatest challenge is to understand what the customer wants. Customers often do not state in so many words what they want, but write things like “I have a flat tire.” From the provided information, the system must recognize that the customer’s bike is defective and that they need assistance.
- Issue of changing data
In order to detect human intention, the AI must recognize a pattern in the available examples. One problem here is the fact that the data keeps changing, for instance due to questions being adjusted, answers being deleted, or new questions and answers being added. To deal with this, the system also needs new examples in order to “learn” from them and recognize a pattern.
- “small data” problem
A further problem is “small data”. There is not always a large number of examples available for the system to learn from. Making manual entries, assigning example queries to a certain type of query, is very costly for companies.
- Filtering relevant information from different texts
Customers often write a long text rather than a short question containing all the relevant words. The system therefore has to filter out those parts of very diverse texts that contain the relevant pieces of information.
How do we deal with these challenges?
This is a task that is very hard to master as it is virtually impossible to develop a system that works perfectly from day one. Even tech giants, such as Google, Apple, and Facebook, find it very difficult to create such an environment. This shows how difficult it is to master this challenge. Our approach is therefore a combination of concept based learning and a loop of continuous data collection (data model loop).
Data model loop
When we talk of the “data model loop”, we mean a loop of continuous data collection without human intervention. Triggered by signals of varying strength in the environment of the customer service staff and customers in the system:
-
The customer service employee answers a question by accessing the database (strong signal)
-
A user types a question into the interactive contact form and clicks on the answer (medium strong signal)
-
Phrases and texts are correlated (not a human signal: weak signal)
In this way, the “small data” problem is overcome and new data is continuously collected to enable the AI to learn new concepts. What we mean by a concept is, for instance, the fact that the technology can make a connection between several questions in order to find the best possible solution for the customer. Through this concept-based training, the AI “learns” without any manual effort by staff.
Conclusion: Shared mobility and customer support
In order to improve the customer service experience, our focus is therefore on recognizing the customer intention. Customers want competent and fast help if problems occur, not waiting 2 - 3 days for an answer. Especially in the rapidly changing mobility industry, customers need an immediate solution to their problem. So that the customer is able to quickly continue driving by car or bike after a breakdown. GPS data, for example, can be passed on via the chatbot. We therefore use artificial intelligence so that all service channels can access the entire knowledge of the database and give the customer a direct and quick answer.
In the background, our AI-based system deals with “small data” and uses the “Data Model Loop” so that new data can be constantly captured, from which the system learns without any manual effort.
This enables us to successfully improve customer service and create a positive customer experience. In the mobility industry, numerous customers use our intelligent technology and answer over 100,000 queries per month with a solution rate of over 50%. We are looking forward to further customers from this sector.
If you would like to learn more about us and our technology or see the software in action, please get in touch :)